With the rising prevalence of data mining, surveillance analytics, and personally tailored user experience within our online navigation, the ways in which we occupy, understand, and engage with our digital footprints becomes a fluid concept—bending and shifting based on the power structures at play “under the hood” of the digital spaces that we occupy.
In response, scholars such as Estee Beck (2015) have worked to expose the ways in which our “visible” digital identities are only a fragment of the footprints we leave behind as users, arguing that the “invisible” digital identity is perhaps just as powerful as the ones we carve out for ourselves. In recognizing the power behind big data, companies have worked to analyze the spaces and choices we make online, subsequently customizing advertisements and anticipating the trends that make something “viral” or successful in the online world.
As a result of thinking through this capitalization on data mining and “invisible” digital identities, I see an inherent connection between digital rhetoric, information literacy, and the ways in which we can push against, or wield these digital analytic tools toward our own ends as both users of the tools and participants of the discourse in the online public sphere.
In order to understand these analytical tools used for data mining and surveillance, we need to think like the machine. To do so, I developed a unit for my Introduction to Digital Technology and Culture class that explored the relationship between human and machine, seeking to push against and challenge our conceptions of what it might mean to anticipate trends in a conversation after the election, gathering data and unpacking its results in a way that requires the collaborative effort of both human and tool. As a result of this cyborg-like process, students ultimately concluded that in any data collection, human interpretation is essential in order to understand the cultural, kairotic, and critical implications of how conversations and discourse live online.
The Assignment
Teaching digital rhetoric during an election year necessarily lends itself toward a conversation concerning the ways in which political discourse lives online. While this assignment asked students to choose a concept that they felt they were an “expert” in within popular culture, given the attention and saturation in the political environment concerning life post-election, over 60% of the topics that students engaged with for this unit dealt with conversations concerning Trump, #BlackLivesMatter, Bernie Sanders, or another topic sensitive to political discourse.
Working with the textual analysis tool, Voyant, the project contained a series of “steps” informed by the Ted Talk by Brian Kennedy on visual literacy. Kennedy offers a methodology for thinking through how we can enhance our visual literacy, arguing that first we must be able to describe what we see, moving toward an ability to analyze, interpret, and subsequently understand the image.
Borrowing this same methodology, I asked students to follow a similar approach in their data collection, visualizing the results of the textual analysis tool Voyant in how predictions about a particular conversation online can be informed by data analysis. Similar to programs such as “Wordle” or “TAPoR”, Voyant is a suite of data visualization tools geared toward unpacking various relationships, frequencies, and occurrences of digital text in a particular corpus.
Whether you are working to analyze one text, or a collection of multiple sources, Voyant’s interface allows for the ability to personalize your relationship to data with the ability to choose from multiple data visualization tools. For example, if you wanted to know how particular words evoked certain relationships among sources, the “knots” data visualization tool would be helpful to conceptualize points of convergence and divergence based on sources engagement with the term.
To help illustrate this assignment, I will move through a topic chosen by a student in the class: Donald Trump’s tax returns. As part of choosing their topic, students had to exhibit their ethos, arguing that they knew the conversation well enough to be able to predict its particular slant online (this student went on to explain their participation in keeping up with Trump’s tax returns at home given their home environment and ties with the IRS).
Next, students developed a prediction about that conversation. This prediction had multiple factors to consider that were dependent on the kairos of the topic, the sources that would be used in Voyant, and the keywords that would subsequently be used to track the prediction.
Returning to our example, the prediction concerning Donald Trump’s tax returns predicted an overall “deceptive” connotation to the conversation, arguing that ultimately, the conversations online concerning this topic would be overwhelmingly skeptical and negative as opposed to supportive or trusting in nature. To track this prediction in Voyant, the student developed a set of five keywords they believed to be the most prevalent in the ten sources they were required to gather, with a justification to accompany both the order and term of that keyword.
To help account for any skewed data, students were also required to create an omissions list for words that might interfere with Voyant’s ability to track the conversation online. This omissions list not only helped to filter prepositions such as “and”, “the” or “on”, but also contained 8-10 words that we discourse-specific. These omission words required a larger understanding of the conversation, and how topics closely related or occurring during the same time might skew the data based on the conversations happening online (topics such as Trump’s cabinet, the immigration policy, and Russia).
Students also found that depending on the sources they chose, they also needed to add words contained within advertisements or the website design itself such as “comment” or “like”. In certain instances, the text within the source had to be stripped of the platform and put into a word document, as the multimedia content hosted on the site interfered with Voyant’s ability to process the textual content.
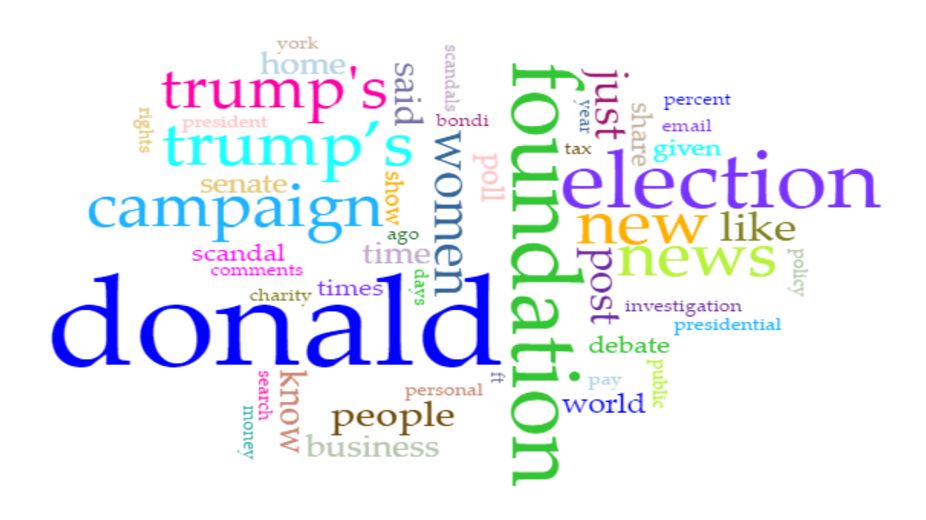
As a result of plugging their ten sources into Voyant, students were able to analyze Voyant’s identification of the top five frequent buzzwords against their own predictions, using the data visualization tools and functions that Voyant offers in to unpack and interpret why they may have been off, correct, or somewhere in the middle. As part of thinking through this data, students presented on their findings in both a presentation and written report, offering up a discussion concerning what its like to work with and think like a machine.
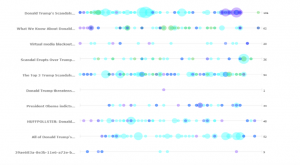
Conclusions
Overwhelmingly, students concluded that data analytics are helpful for thinking through the ways in which conversations manifest, shift, and occur in online spaces, however, they offered the caveat that human cognition and interpretation are essential in unpacking and understanding this information. If we only rely on machines for understanding the ways in which political discourse functions online after the election, we fail to account for how other factors such as how the platform, community, and cultural assumptions concerning that topic inform the ways in which it manifests.
Learning to see data under the framework of data analytics offers students possibilities for understanding a methodology similar to how our “invisible” digital identities are gathered by various companies. While Voyant is predominantly used for analyzing text in a given corpus, the social and cultural implications for tracking online conversation can reveal striking insights into the assumptions we draw concerning a particular conversation, operating similar to the ways in which surveillance analytics monitor conversations in social media spaces.
As such, this assignment concluded with pushing against a dichotomy of “human vs. machine” and ultimately concluded that if we are to be informed users, producers, and investigators of digital culture, we need to approach these conversations as “human with machine”, working together synchronously to draw striking conclusions of how information literacy, digital rhetoric, surveillance, and data analytics continue to take shape and play important roles in a changing political landscape.
References
Beck, E. (2015). The invisible digital identity: Assemblages in digital networks. Computers and Composition, 35, 125-140.